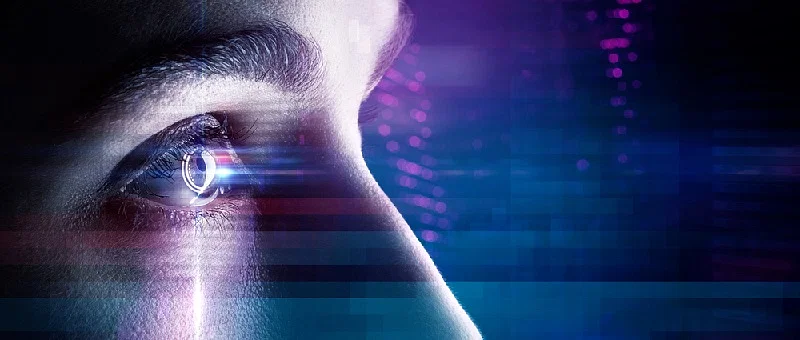
Refractive errors are visual conditions that arise from the eye's ability to correctly focus light. The most common types of refractive errors are nearsightedness (nearsightedness), farsightedness (farsightedness), and astigmatism (distorted vision). These errors can cause problems such as blurred vision, eyestrain, headaches, and difficulty reading or driving.
Refractive errors can be corrected with glasses, contact lenses, or surgery. However, it is also important to detect and treat them early, since they can cause complications such as glaucoma, retinal detachment or blindness.
How can refractive errors be predicted? One traditional way is through an ophthalmological examination that measures the optical power of the eye. However, this method can be expensive, invasive, and subject to human error. For this reason, some researchers have explored the possibility of using machine learning to predict refractive errors from the characteristics of the foveal pit.
The foveal pit is a depression in the center of the fovea, a small area of the retina that is responsible for sharp, detailed vision. The foveal pit contains high concentrations of cone photoreceptor cells, which are responsible for color vision and visual acuity. The shape and size of the foveal pit have been related to refractive errors, with the shallower pits associated with myopia and the deeper pits associated with hyperopia.
A study published in 2020 in the Investigative Ophthalmology and Visual Science journal titled "Machine-Learning Predictor of Refractive Error from Foveal Pit Characteristics" aimed to investigate the potential of machine-learning algorithms to predict much refractive error in foveal pit characteristics. foveal.
The study performed optical coherence tomography (OCT) to capture high-resolution images of the foveal pit in 100 healthy eyes of 50 participants. The images were then analyzed using machine learning algorithms to predict refractive errors. The study found that machine learning algorithms could accurately predict refractive errors in pit features foveal, with a precision of 0.85.
The use of machine learning algorithms to predict experienced refractive errors in foveal fossa features has several potential clinical applications. For example, it could be used in screening programs to identify people at risk of developing nearsightedness or farsightedness at an early stage. It could also be used in personalized treatment planes for people with refractive errors, identifying the optimal treatment strategy based on the characteristics of the foveal pit.
In conclusion, the study highlights the potential of machine learning algorithms to predict refractive errors extremely on foveal fossa features. The results of this study have important implications for the diagnosis and treatment of refractive errors, and more research is needed in the future.
Emiliano Teran
To know more:
Emiliano Teran, Pablo DeGracia, Arturo Yee-Rendon, Abel Ramon, Silvia Paz-Camacho, Carla Angulo-Rojo, Omar Garcia-Lievanos; Machine-Learning Predictor of Refractive Error from Foveal Pit Characteristics. Invest. Ophthalmol. Vis. Sci. 2021;62(8):2153.